8: Looking ahead with evolving learning machines
- InvestEngines
- Sep 1, 2019
- 5 min read
Updated: Mar 31, 2023
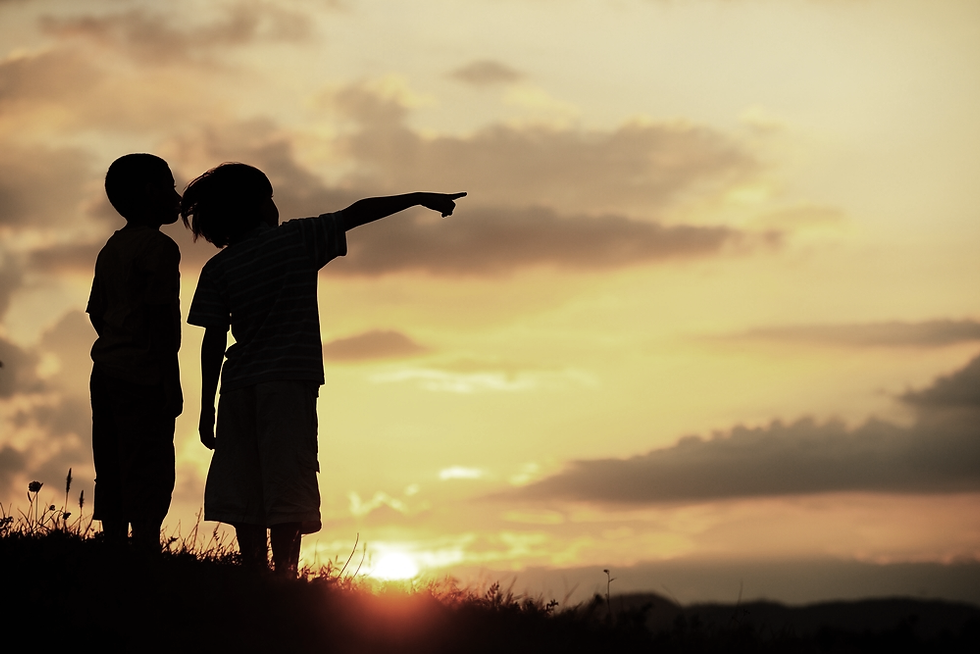
Predicting is a fool's errand and the view from 2020 is anything but certain. Some of the modeling of InvestEngines traverses almost 100 years of data. The specific asset class training window for the benchmark portfolio includes 25 years of data, 1994 until 2019. Since going live with data beyond that training window I am cautiously optimistic about what the machines are capable of, while being cognizant of their limitations and needed improvements.
But when the unexpected happens it is important to revisit your assumptions. The speed and impact of Covid 19 was a major stress test and real time teaching moment for the learning machines. Some of the challenges of machine learning are that any causal relationships that may exist are complex and largely hidden. Furthermore, since the machines learn from the past, there is nothing to guarantee that any future conditions match any of those in the past. And it is fair to say the pandemic presents us with a unique set of conditions.
Over the past 20 plus years, the simplest 2 engine version of InvestEngines has only generated about 1 re-allocation per year on average. The current 3 engine model seems to be the best trade-off between complexity and performance. It is the default version being used to generate signals at a bit over 2 per year, or about every 5 months on average.
But there is a very important difference between those previous periods and now. The tech bubble and the financial crisis negative effects occurred over much longer time frames and interest rates and bond yields were much higher as those crises emerged.
In those instances, the learning machines moved from over-weighting equities to over-weighting bonds that protected on the downside. Then they moved to a more balanced diversification as the recovery continued. The speed of the market's drop and recovery this time has been so fast, so far, the re-allocation moved to a full diversification of equities and bonds without first over-weighting bonds as it did in the past.
If the recovery stalls, we may yet see a shift to over-weighting bonds as the next re-allocation. Bond yields are already at historically low levels, so higher returns from here may be counter intuitive and certainly nothing is assured. The FED has said it has no plans to go negative, but if interest rates do go negative (as they have in Germany), bond funds could still outperform equities as they have over the past 12 months (the long bond has returned 20% over the last 12 months). Time will tell.
There are important limitations in the current InvestEngines data sets that will impact future actions.
Gold
The InvestEngines portfolio is limited and does not include some key asset classes. Precious metals, especially gold, are considered an asset class to hedge against inflation or currency devaluation, which has not been an issue for the past 25 years. In anticipation of a new regime with a renewed impact of precious metals I have built a 3rd competing engine that includes gold. As expected the results were very marginally improved only when used in conjunction with the current models. Gold averaged around 1% asset weighting, never reached more than 13% and is currently weighted at 0%. Gold is a very volatile asset, but worth watching as the future unfolds and the risk of inflation rises. It is now added to InvestEngines modeling as the benefits are sufficient relative to the added volatility and complexity.
Speed
The recent rise in the speed of change is sufficient reason to explore the effects of speeding up the response time of the learning machine. The corona virus negative impact and subsequent speed of the market's recovery so far are unprecedented. A much faster responding component of the learning machines may be in order. Recent testing such a concept resulted in very marginal improvements overall, but more significant benefits when things move as fast as they have during the pandemic. However, the increased response speed didn't help significantly during the pandemic and is not being used in the current reporting model.
Granularity
In the past I explored more granular asset classes, for instance, by country or asset type rather than simply US, developed markets Ex US, emerging markets, aggregate bonds and long bonds as currently used by InvestEngines. But the modeling grows exponentially more complex and the results are similar over the long run. Adding granularity appeared to have reached a point of diminishing returns. But with the pandemic having such profound effects on market sectors it seems that adding granularity of asset classes may be worth reconsidering in the future, including cash.
Conclusion
So what comes next? Let’s start with some qualitative observations.
1. The market seems disconnected from economic realities.
2. Debt to GDP ratios are at all time highs, interest rates are at all time lows
3. A recession officially began this spring.
4. Covid 19 long term effects on our health and economy are still unclear
5. Geo political dynamics are anything but stable
6. Everything seems to be happening faster than ever
7. We are facing socio-economic imbalances and cultural dysfunction
That list is a witch’s brew of uncertainty. In the near term, say the next couple of years, it will take at least 3 things to occur for positive market outcomes: continued monetary support from the FED, continued fiscal support from congress and socially accepted medical solutions for the pandemic. All 3 things happening is a tall order. That the learning machines ignore emotion and the decoupling of economic data relative to market data is both comforting and worrying. And the longer term impact of those things is unclear. Only time will tell.
So what do the “experts” say? The upshot: diversification is key, which is supported by the learning machines' current metrics. But the question remains how much can classical diversification help and what are its limits. That is something that will be explored.
Over the next 5 to 10 years, the historical modeling of the learning machines indicates returns for equities and bonds should be well below their historical averages. There is an 80% chance that average annual equity returns will fall within the range of 2% to 4% per year as shown in the scatter plot below.
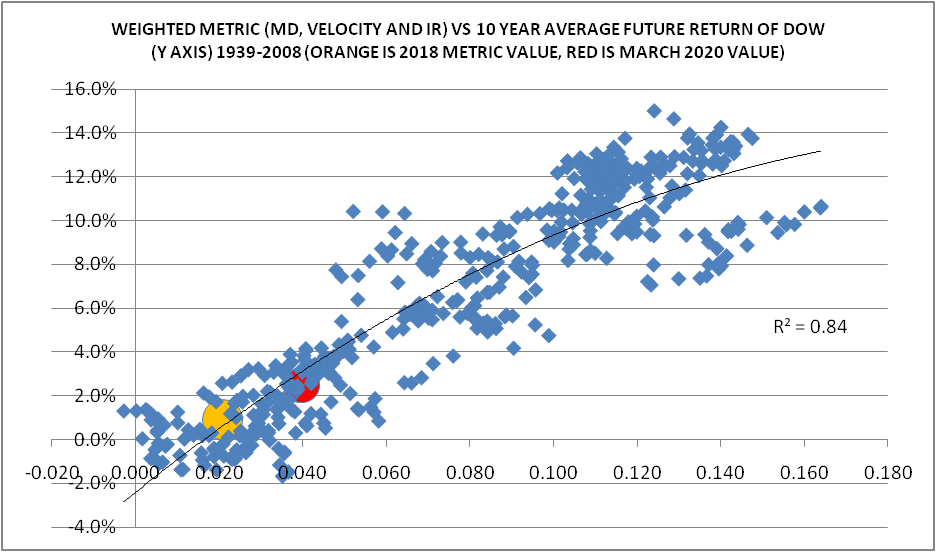
And if we look at the longer term trends (25 years) it appears that there has been a continuous erosion of average returns. Below is a 25 year history (1995-2020) of how a diversified portfolio has performed. It is a graph of the rolling 12 month return of the benchmark portfolio (red) and the learning machine asset allocation portfolio (blue). (The huge negative red blip is the financial crisis, the learning machines, blue line, dramatically reduced that negative spike.)
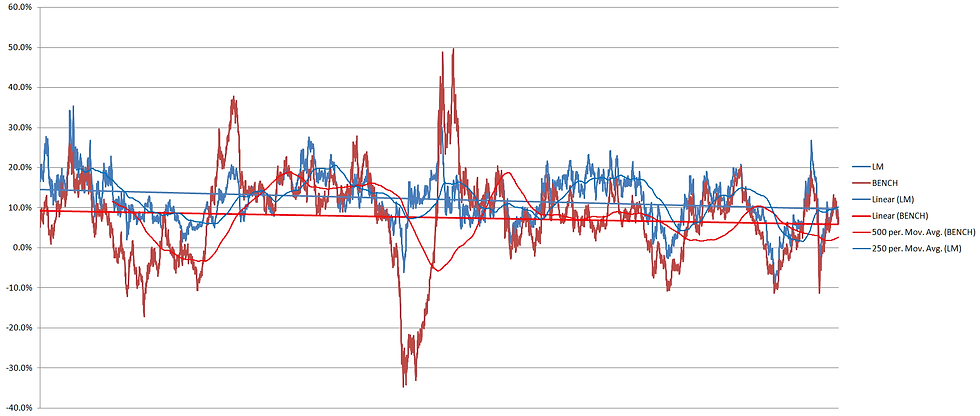
What the above plot shows is that there has been a steady erosion of annual returns. Not only for the benchmark diversified portfolio, but for the ability of the learning machines to improve matters. This is due to the fact that interest rates have been on a glide path towards zero for 25 years. It has been a tail wind for bond returns as well as equity returns. Today with interest rates near zero and with little of hope of decreasing further the best we can hope for is a flattening of that 25 year decline. If interest rates do begin to rise sustainably, that 25 year tail wind, will become a head wind further eroding average returns. And the downward slope of returns will continue.
Predicting a specific 1 year return with any certainty at any point in time is not an accurate exercise. However, for planning purposes, the graph above also includes the rolling 1 year average of 1 year returns for the learning machine and a 2 year rolling average for the benchmark. This can be used as one potential indicator to judge how much cash to have on hand (e.g. need the cash to meet spending obligations without selling investments or as dry powder to add to your investments at market bottoms). If that rolling average approaches zero, you've reached that threshold.
But if you want to take a closer look at what might be possible in terms of near term future expectations, the article titled "climbing further out on a limb" explores shorter term movements in the market.
Comments