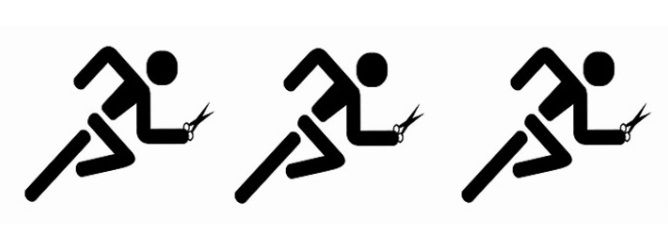
This is an "old" expression for taking unnecessary risks and a metaphor regarding the use of leverage. Its risky business. Leveraged ETF’s are easy to use, but the leverage comes from borrowing. And they reset every day, so the daily results are neither symmetrical nor cheap. The borrowing costs mean up days are "up less" than down days are down. Volatility will hurt big time. Major draw-downs will be more damaging than up trends will be beneficial. And fees are higher. These are high risk funds and I’ve been hesitant to consider them.
So why even investigate such a thing for long term investors? I wanted to explore two questions: Is it even possible to improve long term returns with leverage and if so, what’s the “price”? Leverage reminds me of the poem by Henry Wadsworth Longfellow "There was a little girl"-
There was a little girl,
Who had a little curl,
Right in the middle of her forehead.
When she was good,
She was very good indeed,
But when she was bad she was horrid.
Just plugging in levered funds into a 60/40 portfolio and hope for the best doesn’t work over the long run. It generates a little pain in flat markets, it delivers serious pain in down markets, but dramatic increases in up markets.
It turns out a learning machine may improve matters. (In a parallel experiment, I have also explored the use of “short” ETFs as well, but the statistics were not encouraging and I have abandoned that work for now.)
Here are the numbers comparing a 60/40 portfolio, to an AI guided active allocation portfolio with the same assets and a third version of that portfolio replacing some assets with broad sector levered funds. Over the past 20 plus years one can expect a typical 60/40 diversified portfolio to return about 8% per year on average. Using the learning machines of InvestEngines to determine active asset allocations, average returns can be raised to about 12% per year. With the learning machines for asset allocation and the stop loss engines for the levered funds, long term average annual portfolio returns may be raised close to 30%. But be prepared to accept a worst case portfolio peak to trough of -50%. (Stop loss learning machines are needed to individually protect from much worse drops of the leveraged funds to achieve this, something that non-leveraged funds don’t require.)
The simple approach I took was to include two 3X levered macro funds (a levered long bond fund and a levered US equity fund) in a portfolio. There were 2 hurdles to overcome. These levered funds have histories of less than 10 years and major peak to trough drops can be catastrophic (-80% to -90% worst case peak to trough). The solution was to synthesize longer term performance to generate training data from their actual tracking index and to use stop loss learning machines to reduce the negative performance of major draw downs. The final step was to apply the allocation engine and stop loss engine to this new portfolio.
It is worth dissecting the performance over 20 years in each of the periods of constant allocation to reveal greater insight. The data is shown in the table below. Note that the average period of constant allocation ranged from 1.5 to 2 years. The average return in the table is the average total return for each of those allocation periods. It becomes clear that 35% of the time the engine was fully levered, and that was when massive (145% on average) gains were generated.
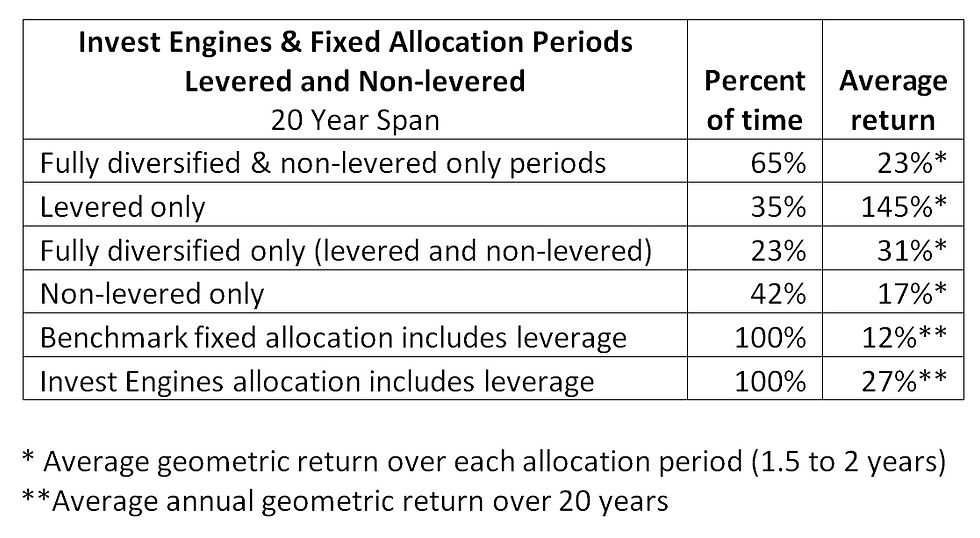
Another view into the effect of leverage is to look at the cumulative net excess of the non levered portfolio to the levered version. That is shown below the periods when the learning machine included periods of leverage and out performed the non-levered portfolio are apparent. This data is taken over the training window, so there is plenty of uncertainty about what comes next. Although the persistent periods of the benefits are compelling.
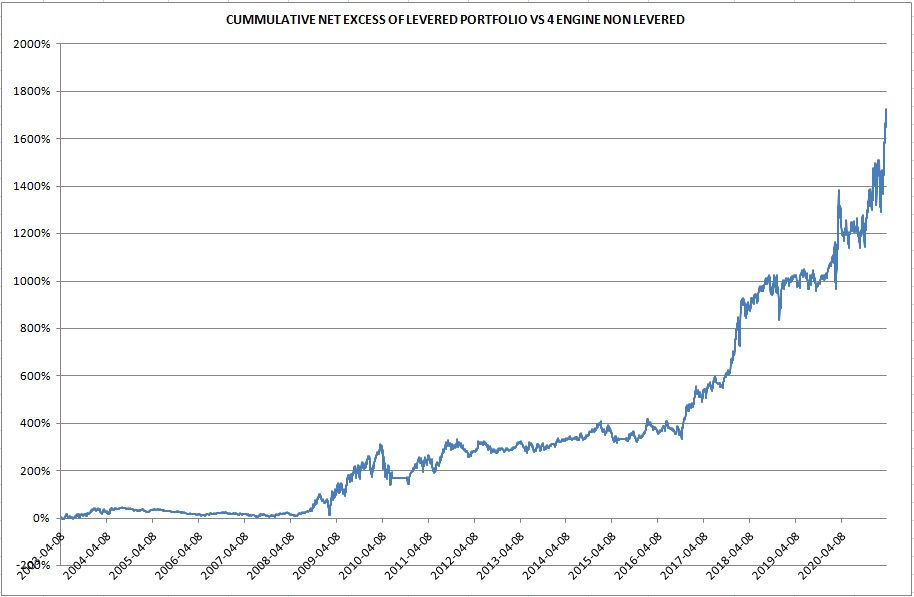
The next step was to see if competing or combining the 4 engine non levered learning machines with the levered learning machine could find a better outcome. Assuming once again, that the highest utility function (RSQ) is the best solution, it turned out that a competing method could deliver higher returns, but with higher volatility and a lower RSQ. However, by simply weighting the two approaches the overall RSQ could be raised above the less volatile non-levered portfolios, or kept the same with higher average returns, which is what I've selected as the optimal solution. I found this to be the most surprising outcome and never expected to be able to improve RSQ with leverage, or to improve long term returns without sacrificing RSQ.
Below is a summary of those results. The bar charts are the probability distributions for the average annual returns of each of the 3 approaches: The levered portfolio learning machine, the standard 4 engine learning machine, and the weighted average of both, respectively. The average annual returns for each approach over a 20 year training window , are respectively, 30%, 12%, and 18%. The line chart below the bar charts shows the historical performance of each approach and their associated RSQ values.
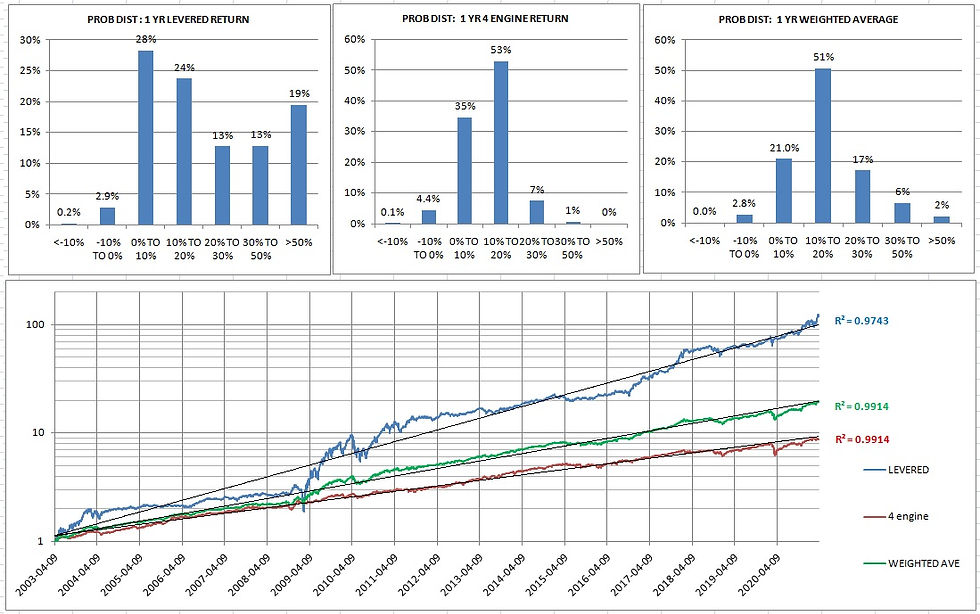
As of this writing, September 1, 2023, the allocation engine suggests 100% of its weight in non-levered US equities (no bond holdings, no levered holdings). That most recent allocation signal occurred in May of 2022 and the most recent 12 month return was 10.8%. The InvestEngines allocation engine for the non-leveraged benchmark portfolio is fully diversified currently and its most recent 12 month return was 5.2%. The benchmark 60/40 fixed allocation portfolio reported a 12 month return of 1.3%.
I will be considering whether to include the levered portfolio as well as the currently reported benchmark 60/40 portfolio, the InvestEngines allocation portfolio and a combination of levered and non-levered portfolios in future updates. When the learning machine indicated to go all in on leverage it made a marked difference. But leverage can be very risky, like running with scissors.
Comments