11: Artificial Intelligence is growing and available as machine managed ETFs
- InvestEngines
- May 20, 2019
- 3 min read
Updated: Dec 4, 2022
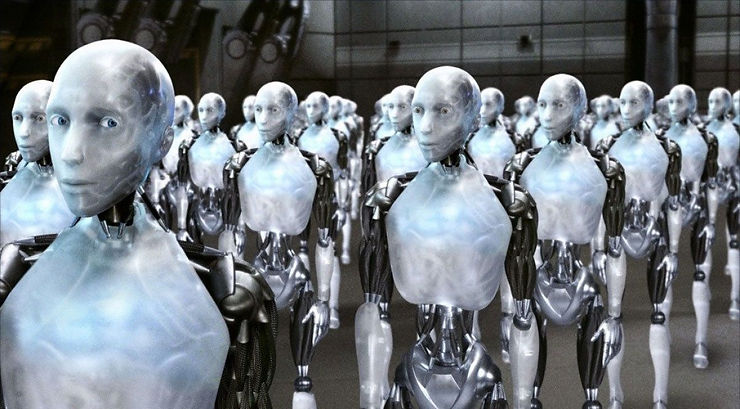
A few startups (e.g. Equbot in 2017 and Qraft in 2016) are focused on using Artificial Intelligence (AI) to make investment choices and construct portfolios. They have made progress and provide easy access to the output of their respective learning machines in the form of machine managed ETFs. No surprise, as I expect an avalanche of such companies and products. It will be important to understand their focus, approach and potential added value for InvestEngines.
This is early in the evolution of businesses and products like these and more are surely on the way. They do share the vision that data is critical to train the learning machines. And to that end these new enterprises are dealing with much larger data sets, processing power and modeling complexity than anything underpinning InvestEngines. I would expect improvements in a number of areas that could benefit InvestEngines by simply including these ETF’s into new portfolios.
But I am always wary of the “new”, there is no free lunch and there are always tradeoffs to understand. The long term training windows InvestEngines used to uncover broad predictive patterns of interest rates and equity valuation go back 100 years. The more granular asset class training data included three market dislocations that are critical to stress test any AI: the tech bubble in 2000, the financial crisis in 2007/2008 and the pandemic of 2020.
Another important matter to understand is; what is the “utility function” of the AI (or how to judge that an AI is being successful). The utility function is critically important in that it must align with what is most important to the user. The first iteration of InvestEngines focused on maximizing total return. That turned out to be a less than ideal choice. Although seemingly obvious, it didn’t always align with a long term investor’s goals. It could be misled by its own success (e.g. avoiding sequence risk) as well as being blind to its own failure (viz. nothing always works). The second generation of InvestEngines has attempted to avoid those pitfalls and focuses on long term and consistent benefit relative to a benchmark and to accomplish those end goals without excessive turnover.
The next step is to see how these AI managed ETFs compare to InvestEngines and how they might fit inside a new portfolio to add value. The first challenge to face is the fact that these new ETFs have only been in existence since 2016/2017. Based on the work in InvestEngines, it may take at least 10 years of data to generate reasonably representative outcomes for long term evaluation, so more time may be needed to fully access these ETFs. Finally, these new AI driven ETFs currently have a narrower focus on specific asset class targets, rather than the broadly diversified focus of InvestEngines. But I am sure many more ETFs are on the way that will broaden asset class selection.
When the first iteration of InvestEngines was used by professionals, those money managers built portfolios with individual stocks and funds. The thing that struck me was how the learning machines tended to converge on similar long term outcomes, regardless of the portfolio components. The portfolios were all reasonably diversified, but with many different funds and stocks selected by each manager. That led me to focus on a simple, classically diversified, portfolio to construct a benchmark that would be used to train the newer learning machines. There may be an opportunity to use these new ETFs in a similar fashion. But as a lifelong skeptic, sufficient transparency, history and data is missing from these offerings to make a judgement. The jury is still out and will be until many years go by.
コメント